Goodstart Early Learning a finalist in health and safety excellence awards
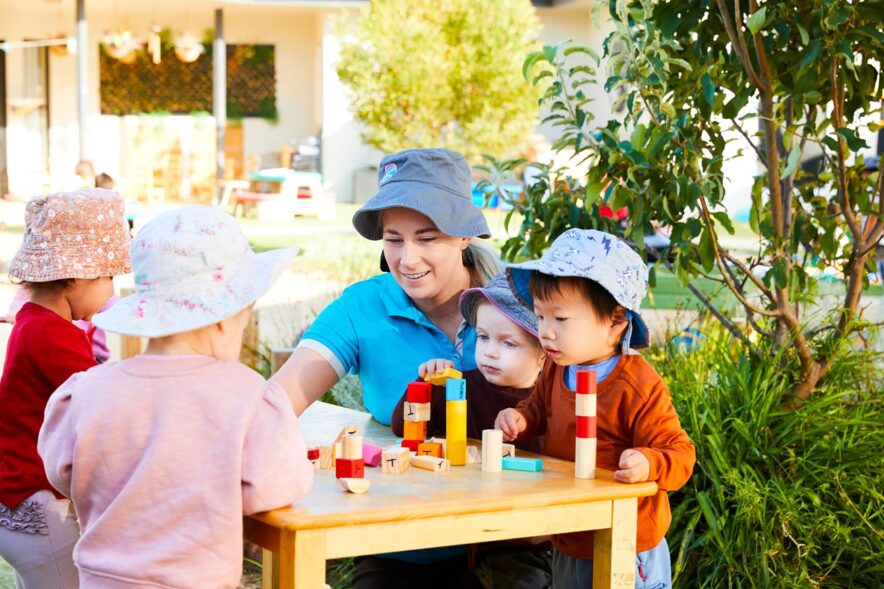
Goodstart Early Learning has been named as a national health and safety finalist for the highly coveted Australian Workplace Health and Safety Awards.
In 2021, the provider took out the national Leadership and Culture award category for its COVID-19 response and this year, its innovative application of machine learning is short-listed for the Large Enterprise: Health and Safety Excellence award category.
Machine learning, a form of artificial intelligence, has been used extensively across the Goodstart network, with Chief Safety Officer Matthew Little saying it has “transformed Goodstart’s way of working.”
The introduction of machine learning in areas such as preventative planning, operational assurance and child and adult injury rates has offered the provider another tool to make its workplaces safer for children and adults.
Over the past 24 months, Goodstart introduced machine learning to improve its safety performance and reduce child and employee injuries, led by Martin Knell, Head of Data and Analytics.
“One challenge with traditional safety statistics is the difficulty to connect injury events with a root cause. For example, traditional statistics often identify slips, trips and falls as the mechanism for an injury but fall short of identifying the patterns and circumstances that led to this,” Mr Knell shared.
Inspired by global perspectives
To identify the most effective machine learning method, Goodstart’s data and analytics team keep abreast of current working theory and took inspiration from international data science competitions that in recent years have featured Gradient Boosting Machines and Neural Networks as winning algorithms for a variety of challenges.
“Not only did we want to create a predictive model, but also understand the important drivers that led to that prediction and selected Gradient Boosting Machines as the algorithm of choice,” he continued.
The machine learning model was trained using 18 months of historic injury data and over 300 parameters. With approximately 70,000 children across the Goodstart network, who collectively spend over 10 million days with the provider each year there is a 50 to 100 times larger “population” than what most university researchers have available to them, and it is this kind of ‘Big Data’ that machine learning really excels on.
Forecasting risk
The accuracy of Goodstart’s predictive model is 98 per cent on a population level and 86 per cent on a decile level. That means, on the test data set, Goodstart’s model could forecast network wide, across more than 660 centres, annual critical child injury numbers with only 2 per cent error.
In real terms, for Mr Little this means there is now a clear map of safety risk for every centre within its 660 plus centre network.
“We have identified where more focus and support is required, we’ve identified great practice, and all the key insights about controllable and non-controllable parameters for child and adult injuries are now front-of-mind – driving the appropriate focus on things that matter most to reducing injury risk.”
.
“While Goodstart’s safety performance is one of the best in our sector we’re always seeking to be better. Improved safety performance is an everyday, and every team member priority.”
Competition for the Awards is fierce, and Mr Little, while hopeful of securing consecutive wins, said to be shortlisted is “reward enough, because it recognises Goodstart’s contemporary approach to health and safety management and that we’re setting new benchmarks.”
Winners of the Australian Workplace Health and Safety Awards will be announced late September.
Popular

Policy
Practice
Quality
Provider
Research
Safety starts with supervision: responding to real risks in ECEC
2025-07-07 10:30:58
by Fiona Alston

Policy
Provider
Practice
Quality
Jay Weatherill appointed to co-lead urgent review into childcare safety in Victoria
2025-07-07 07:24:04
by Fiona Alston

Quality
Practice
Provider
Workforce
Reclaiming Joy: Why connection, curiosity and care still matter in early childhood education
2025-07-09 10:00:07
by Fiona Alston