Diagnosing anxiety and depression in children made easier with AI
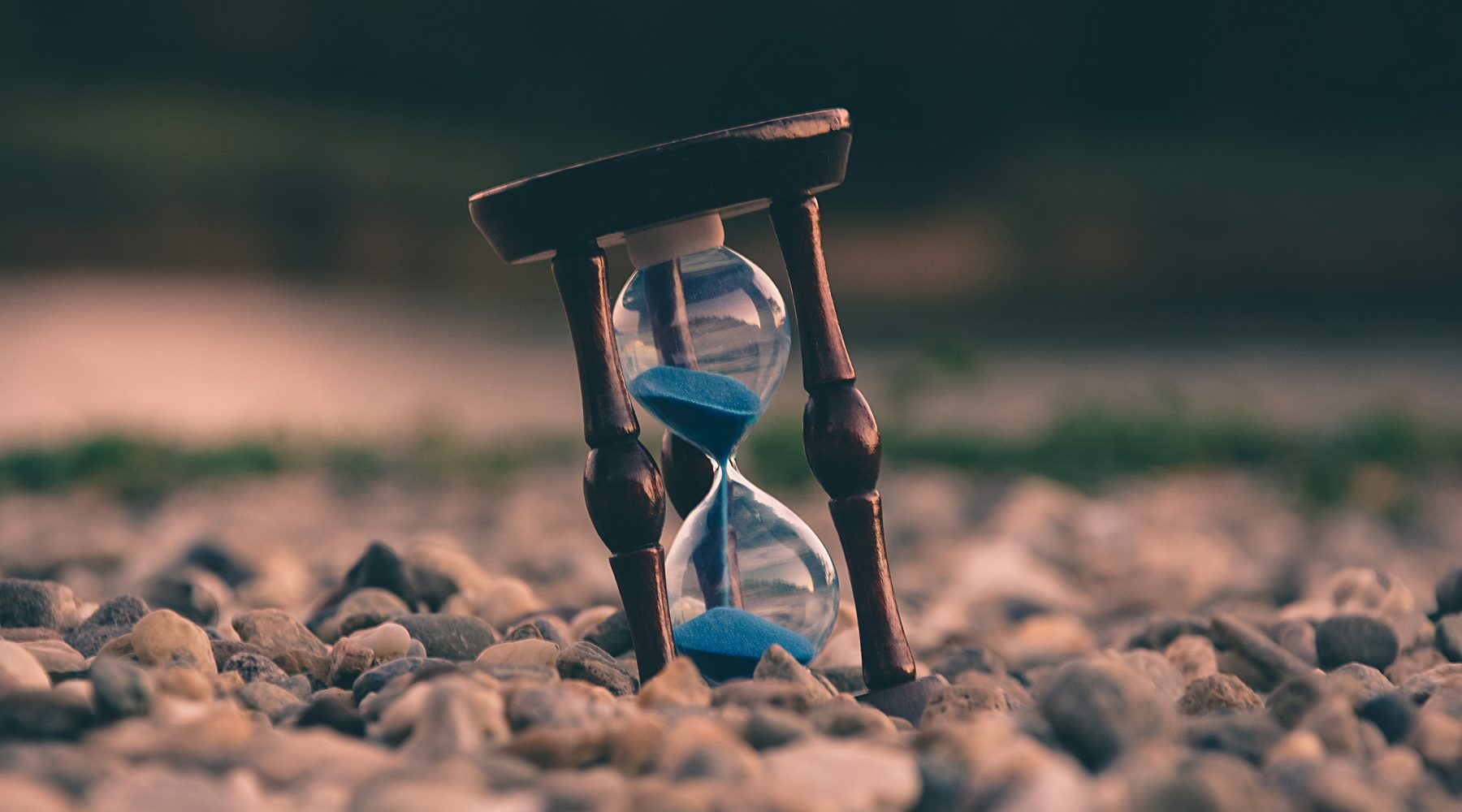
A machine learning algorithm which can detect signs of anxiety and depression in the speech patterns of young children offers a potential solution to the often challenging situation of diagnosing depression and anxiety in children who could be best supported through early intervention, researchers from the University of Vermont have found.
Depression and anxiety can have debilitating life-long effects on children. Around one in five children suffer from internalising disorders (anxiety and depression), but because children under the age of eight can’t reliably articulate their emotional suffering, adults need to be able to infer their mental state, and recognise potential mental health problems, supporting the child with strategies to manage their condition. Waiting lists for appointments with psychologists, insurance issues, and failure to recognise the symptoms by parents all contribute to children missing out on vital treatment.
The research, which has been published in the Journal of Biomedical and Health Informatics, was undertaken because of the need to source “quick, objective tests to catch children when they are suffering,” said lead author Ellen McGinnis.
Ms McGinnis noted that the majority of children under eight with internalising disorders are undiagnosed, and that early diagnosis “is critical because children respond well to treatment while their brains are still developing, but if they are left untreated they are at greater risk of substance abuse and suicide later in life”.
The standard diagnostic process for internalising disorders involves a 60-90 minute semi-structured interview with a trained clinician and their primary care-giver. Ms McGinnis, along with study senior author Ryan McGinnis, has been looking for ways to use artificial intelligence and machine learning to make diagnosis faster and more reliable.
To undertake the research, study authors used an adapted version of a mood induction task called the Trier-Social Stress Task, which is intended to cause feelings of stress and anxiety in the subject. A group of 71 children between the ages of three and eight were asked to improvise a three-minute story, and told that they would be judged based on how interesting it was.
The researcher acting as the judge remained stern throughout the speech, and gave only neutral or negative feedback. After 90 seconds, and again with 30 seconds left, a buzzer would sound and the judge would tell them how much time was left.
“The task is designed to be stressful, and to put them in the mindset that someone was judging them,” Ms McGinnis said.
The children were also diagnosed using a structured clinical interview and parent questionnaire, both well-established ways of identifying internalising disorders in children.
The researchers used a machine learning algorithm to analyse statistical features of the audio recordings of each child’s story and relate them to the child’s diagnosis. They found the algorithm was highly successful at diagnosing children, and that the middle phase of the recordings, between the two buzzers, was the most predictive of a diagnosis.
“The algorithm was able to identify children with a diagnosis of an internalising disorder with 80 per cent accuracy, and in most cases that compared really well to the accuracy of the parent checklist,” said Mr McGinnis.
The speed with which the algorithm operated was also pleasing to the researchers, requiring just a few seconds of processing time once the task is complete to provide a diagnosis.
The algorithm identified eight different audio features of the children’s speech, but three in particular stood out as highly indicative of internalising disorders: low-pitched voices, with repeatable speech inflections and content, and a higher-pitched response to the surprising buzzer.
Ms McGinnis said these features fit well with what you might expect from someone suffering from depression. “A low-pitched voice and repeatable speech elements mirrors what we think about when we think about depression: speaking in a monotone voice, repeating what you’re saying.”
The higher-pitched response to the buzzer is also similar to the response the researchers found in their previous work, where children with internalising disorders were found to exhibit a larger turning-away response from a fearful stimulus in a fear induction task.
The previous work of the researchers involved inducing a fear, and required a darkened room, toy snake, motion sensors attached to the child and a guide. The newly developed voice based assessment needs only a “judge”, a way to record speech, and a buzzer to interrupt. Something, researchers said, which is “much more feasible to deploy”.
Next steps in advancing the research findings will be to develop the speech analysis algorithm into a universal screening tool for clinical use, perhaps via a smartphone app that could record and analyse results immediately.
The voice analysis could also be combined with the motion analysis into a battery of technology-assisted diagnostic tools to help identify children at risk of anxiety and depression before even their parents suspect that anything is wrong.
Popular

Workforce
Quality
Four Australians recognised for services to ECEC and children in Australia Day 2025 Honours List
2025-01-28 07:14:09
by Jason Roberts

Provider
Workforce
Practice
Marketplace
Economics
Rare opportunity to be a part of Explore & Develop with existing service for sale
2025-01-28 02:52:32
by Freya Lucas

Quality
Provider
Jobs News
Practice
Workforce
Fair Work Ombudsman takes legal action against ECEC service operator
2025-01-29 11:21:09
by Freya Lucas